What is Generative AI vs Predictive AI : 5 Best Key Benefits
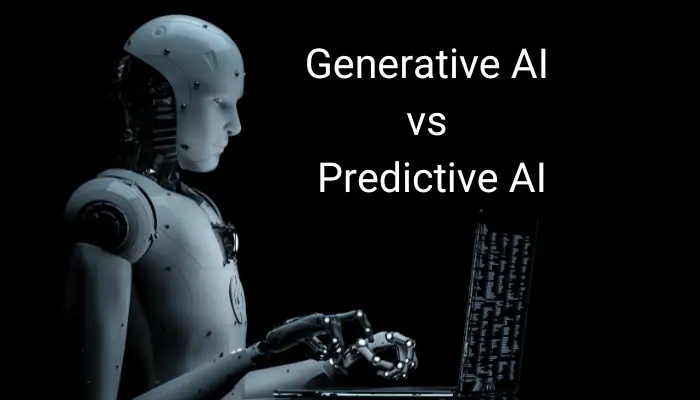
In the context of Generative AI vs Predictive AI, Generative AI primarily focuses on creating new and original content by learning from existing data patterns. It is valuable in creative fields and novel problem-solving. On the other hand, Predictive AI uses patterns in historical data to forecast future outcomes or classify future events. It aids in decision-making, and strategy formulation, and provides actionable insights. Both approaches have distinct applications and benefits within the field of AI.
Generative AI vs Predictive AI: How Does It Work?
Indeed, predictive AI can be seen as a powerful analytical tool that excels in working with numerical data, while generative AI is often associated with creativity, such as writing or creating artwork. By combining both approaches, enterprises can leverage structured and unstructured data to derive value and gain deeper insights.
Generative AI vs Predictive AI
What is generative AI?
Generative AI models go beyond traditional machine learning by incorporating various forms of machine learning systems, algorithms, and neural networks. This enables generative AI to explore the realm of creativity with a tech-based approach.
Some of the algorithms used in generative AI, pioneered in the mid-2010s, include Generative Adversarial Networks (GANs), Variational Autoencoders (VAEs), and Recurrent Neural Networks (RNNs).
- Generative Adversarial Networks (GANs): Used to create images, synthesize voices, and translate voices and videos into different languages.
- Variational Autoencoders (VAEs): Primarily used for generating structured data.
- Diffusion models: Applied to generate imagery and videos in response to prompts.
- Large Language Models (LLMs): Particularly adept at text generation, but newer multimodal approaches allow for tasks like image creation, code writing, robot programming, and automation in response to text prompts.
What is predictive AI?
Predictive AI leverages historical data to identify patterns and make predictions, providing valuable insights for business decision-making. Its ability to detect data flow anomalies and extrapolate their future impact on results or behavior demonstrates its value in understanding and anticipating future trends and outcomes.
Predictive AI utilizes a wide range of machine learning and AI algorithms, including regression, decision trees, random forests, support vector machines, and neural networks.
- Neural networks for a range of tasks.
- Linear regression to identify correlations.
- Logistic regression for data classification.
- Support vector machines for data classification.
- Decision trees for outcome estimation.
- Random forests for data classification.
- K-means clustering for data grouping.
- Self-trained naive Bayes classifier for data classification.
Key Differences Between Generative AI vs Predictive AI
Indeed, there is a fluidity between generative AI and predictive AI, and they share common elements. Their applications and outcomes differ based on how they are utilized. As AI continues to evolve, both generative AI and predictive AI will contribute to shaping the future and have a significant impact on various domains and industries.
Purpose and Goals:
Generative AI aims to produce creative and novel outputs resembling human patterns, while predictive AI focuses on analyzing data patterns to forecast potential outcomes or trends. They have distinct purposes and objectives.
Input and Output Requirements:
Generative AI relies on an initial input, such as a prompt or seed, to initiate the creative process and generates new content based on that input. In contrast, predictive AI utilizes historical data as input to make predictions or forecasts about future outcomes. The output of generative AI is creative content, while predictive AI provides forecasts or predictions based on data analysis.
Training Data and Model Architectures:
Generative AI systems employ techniques such as neural networks, generative adversarial networks (GANs), and reinforcement learning to learn patterns from training data and generate creative outputs. Predictive AI, on the other hand, utilizes statistical algorithms and machine learning models to analyze data and identify patterns for predicting future outcomes.
Benefits: Generative AI vs Predictive AI
Generative AI vs Predictive AI
Generative AI offers significant benefits to content creators, leveraging its vast creative potential. On the other hand, businesses proficient in predictive AI can gain advantages such as understanding future trends and extracting maximum value from their data repositories. Both approaches offer unique advantages in their respective domains.
Benefits of Generative AI: {generative AI vs predictive AI}
- Automates code generation and software development tasks.
- Simplifies the generation of new content.
- Summarizes complex documents or data sets.
- Works effectively with unstructured data.
- Generates articulate and concise responses to complex queries.
- Excels in working across various modalities like text, video, audio, robot instructions, and different data formats.
- Automation of creative workflows, accelerating high-quality output.
- Application in design, art, and entertainment, providing innovative solutions and expanding creative boundaries.
- Proficiency in generating coherent text and images, supporting writing, translation, and artistic endeavors.
- Contribution to data augmentation, personalization, and accessibility, making it versatile across industries like healthcare and finance.
Benefits of predictive AI: {generative AI vs predictive AI}
- Automation of analytics processes.
- Simplification of complex analysis tasks.
- Streamlining of data processing.
- Effective utilization of structured data.
- Improved analysis for well-defined use cases.
- Proficiency in working with structured and time series data.
- Providing insights on future trends, opportunities, and threats, aiding in management decision-making.
- Recommending products, upselling, enhancing customer service, and optimizing inventory levels.
- Adding depth and accuracy to management processes.
- Enabling organizations to extract more value from data by offering detailed information about different data segments.
- Enhancing predictions and improving overall decision-making processes within organizations.
Limitations: Generative AI vs Predictive AI
Generating high-quality content consistently can be a challenge, resulting in occasional outputs that may not meet expectations. Training generative models require significant computational resources and large datasets, making it resource-intensive. Balancing creativity and control, and addressing issues like bias and diversity, remains an ongoing challenge in the development and deployment of generative AI models.
- Propensity to hallucinate.
- Heavy carbon footprint.
- Can be expensive to retrain models.
- Difficult to remove sensitive data once it’s baked into a model.
- More challenging to explain or interpret the mechanisms underpinning results.
Limitations of Predictive AI
Predictive AI’s effectiveness is highly dependent on the availability and quality of data. Incomplete, inaccurate, or biased training data can lead to flawed predictions. Many predictive models, particularly complex ones like deep neural networks, lack interpretability, making it challenging to understand the reasoning behind predictions or identify potential biases in the decision-making process.
- Bias in underlying data might be amplified
- Overreliance on historical data
- Lack of interpretability can arise in complex predictive models
- Overfitting and Struggle with causation
- Too much training on data and business cases can lead to prediction bias
Use Cases: Generative AI vs Predictive AI
Generative AI vs Predictive AI
Use Cases: Generative AI
1. Churn Prevention
Predictive analytics models aid in preventing customer churn by analyzing dissatisfaction among existing customers and identifying at-risk customer segments. Businesses can use predictive data to make necessary modifications, ensuring customer satisfaction and protecting revenue.
2. Customer Lifetime Value
Using predictive analytics to analyze customer data allows businesses to optimize their marketing strategies and target customers with the highest lifetime value, resulting in increased customer acquisition and long-term success.
3. Customer Segmentation
Customer segmentation involves grouping customers based on shared traits or characteristics. Businesses determine their market segments based on aspects that provide the most value to their company, products, and services, allowing for targeted and personalized marketing strategies.
4. Healthcare
Generative AI has significant applications in healthcare, including medical imaging, drug discovery, and personalized treatment plans. It can generate realistic medical images, facilitating precise diagnoses and surgical planning. Additionally, generative AI models can simulate molecular structures and interactions, accelerating the drug design process.
4. Predictive Maintenance
Maintaining cost efficiency is crucial for increasing revenue in businesses. Organizations with substantial investments in equipment and infrastructure face challenges in managing capital outlay. Predictive maintenance machine learning techniques can help address these challenges by optimizing maintenance schedules and reducing unplanned downtime.
- Text: Generate credible text on various topics, including business letters, rough drafts of articles, and annual reports.
- Images: Output realistic images based on text prompts, create new scenes, and simulate new paintings.
- Video: Automatically compile video content from text and create short videos using existing images.
- Music: Analyze a music catalog and generate new musical compositions.
- Product design: Generate multiple possible changes for a new version of a product based on inputs from previous versions.
- Personalization: Personalize user experiences, such as providing product recommendations, tailored experiences, and new material that closely matches their preferences.
Use Cases: Predictive AI
Predictive AI offers numerous use cases across various industries. It enables financial forecasting, aiding in making informed decisions. It enhances fraud detection by identifying suspicious patterns and behaviors. In healthcare, it supports disease prediction and personalized treatment. In marketing, predictive AI helps optimize campaigns and target customers effectively.
1. Finance and Banking:
Predictive AI enables risk assessment by analyzing transaction patterns and credit histories, improving fraud detection and prevention. It also optimizes loan approval processes and portfolio management, enhancing decision-making and efficiency in financial operations.
Predictive AI also enhances financial forecasting by leveraging a broader dataset and correlating financial information with other forward-looking business data, leading to improved accuracy in predictions.
2. Manufacturing and Supply Chain Management:
Predictive AI analyzes real-time data from sensors and monitors, enabling the anticipation of equipment failures before they happen. This proactive approach minimizes disruptions, improves productivity, and reduces maintenance costs, making it highly valuable in the manufacturing and supply chain industries.
3. Fraud detection:
In fraud detection, predictive AI can identify potential fraud by detecting anomalous behavior. For instance, in the banking and e-commerce sectors, unusual device usage, location, or requests that deviate from a user’s normal behavior can raise suspicions. An example would be a login from an unfamiliar IP address, which serves as a clear warning sign.
Understanding the capabilities of both generative vs predictive AI empowers us to leverage their benefits while responsibly addressing ethical considerations that arise in their use.
What is predictive AI vs generative AI vs machine learning?
Predictive AI focuses on forecasting future outcomes using historical data patterns, while generative AI creates novel content like text, images, or music. Machine learning is a broader concept that encompasses both predictive and generative AI, referring to algorithms that improve performance through experience and data.
What is Generative AI vs Predictive AI : 5 Best Key Benefits