PyTorch: Transfer Learning vs Fine Tuning| 2 Best Techniques
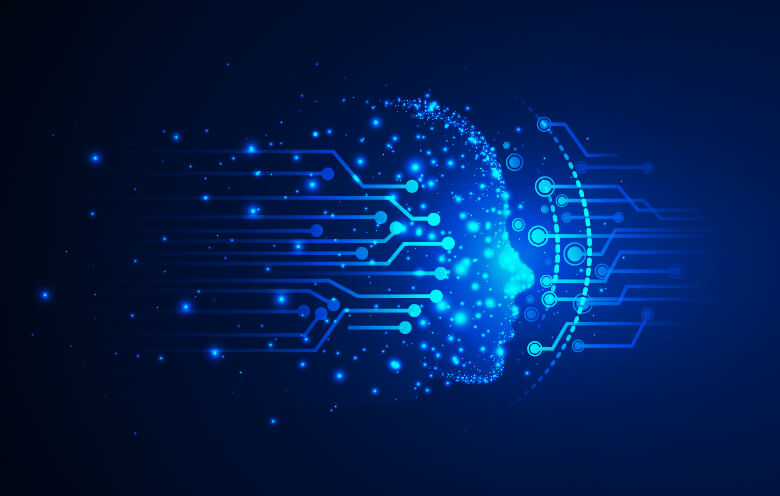
Transfer Learning vs Fine Tuning: In the field of deep learning, transfer learning and fine-tuning are two powerful techniques that enable the leveraging of pre-trained models for new tasks. Deep learning has revolutionized the field of machine learning, enabling remarkable advancements in various domains. Two key techniques that have greatly contributed to this progress are transfer learning and fine-tuning.
While transfer learning and fine-tuning share the concept of utilizing pre-trained models, they differ in the extent of adaptability. Transfer learning primarily focuses on training a new task-specific layer on top of fixed, pre-trained layers, providing a head start in learning representations. On the other hand, fine-tuning offers more flexibility by allowing the modification of earlier layers, enabling the model to refine its learned features for the target task. Fine-tuning strikes a balance between leveraging general features and customizing them to the specific task.
In this blog post, we will explore the concepts of transfer learning and fine-tuning, highlighting their similarities, differences, and practical implications.
Overview: Transfer Learning vs Fine Tuning:

Transfer learning involves taking a pre-trained model and using its learned features as a starting point for a new task. It involves freezing the initial layers and training only the top layers on the new dataset.
Fine-tuning, on the other hand, involves unfreezing some of the earlier layers of the pre-trained model and training them along with the top layers on the new dataset to adapt the model to the new task. Fine-tuning allows for more flexibility and customization compared to transfer learning alone.
In the context of transfer learning vs fine tuning, transfer learning is a machine learning technique where knowledge gained from training one model on a specific task is transferred and applied to another related task. It allows pre-trained models to be utilized as a starting point for new tasks, enabling faster and more accurate training with limited data.
Transfer learning involves leveraging knowledge gained from pre-trained models trained on large-scale datasets and applying it to new, related tasks. Instead of starting from scratch, transfer learning allows us to kick-start our models with pre-learned features and representations. By reusing the learned weights, we can benefit from the generalization capabilities of the pre-trained models, especially when the target task has limited training data. Transfer learning serves as a powerful tool for accelerating model development and achieving better performance with reduced computational resources.
Transfer of learning types: {transfer learning vs fine tuning}
Fine-tuning is a process in transfer learning where a pre-trained model is further trained on a new dataset specific to the target task. By adjusting the model’s parameters based on the new data, it can adapt and specialize its knowledge to perform better on the specific task, improving its accuracy and performance.
While transfer learning provides a head start, fine-tuning takes it a step further by adapting the pre-trained model to the specific nuances of the target task. Fine-tuning involves adjusting the weights of the pre-trained model by introducing a smaller task-specific dataset. By updating the model’s parameters, we allow it to learn task-specific features and improve its performance on the new task. Fine-tuning strikes a balance between leveraging the pre-trained knowledge and tailoring the model to the specific requirements of the target task.
What is fine-tuning in transfer-learning Python?
Fine-tuning in transfer learning, in Python, refers to the process of taking a pre-trained model from a deep learning library like TensorFlow or PyTorch and adapting it to a new task by updating its weights using a smaller task-specific dataset. This allows the model to learn task-specific features and improve performance on the new task.
Fine-tuning in transfer learning refers to the process of taking a pre-trained model, usually trained on a large dataset, and further adjusting its parameters on a smaller, task-specific dataset. This allows the model to adapt its learned representations to the new task, improving its performance and accuracy.
Transfer Learning vs Fine Tuning: What’s the Difference?
Transfer Learning and Fine-Tuning is often used interchangeably, but it has distinct meanings and purposes when it comes to learning a new task.
Transfer learning involves leveraging a pre-trained model as a knowledge base to address new but similar problems. It captures general patterns and features from a dataset and applies them to the new task. By building upon the pre-existing knowledge, transfer learning significantly speeds up the training process and improves performance on the new task.
Fine-tuning, on the other hand, focuses on adapting a pre-trained model to a specific task by further training it on a task-specific dataset. Rather than starting from scratch, fine-tuning allows for the adjustment and optimization of the model’s parameters to better align with the target task. This process refines the model’s learned features and enhances its ability to perform well on the specific task at hand.
Transfer Learning vs Fine Tuning: What Experts Say